AI Algorithm Spots Abnormal Chest X-Rays With 90% Accuracy
- Thomas Dworetzky, DotMed.com
- Sep 20, 2018
- 3 min read
AI algorithm qXR from healthcare startup Qure.ai can spot chest X-ray abnormalities with 90 percent accuracy, according to a study appearing online on Cornell University's arXiv.org site.
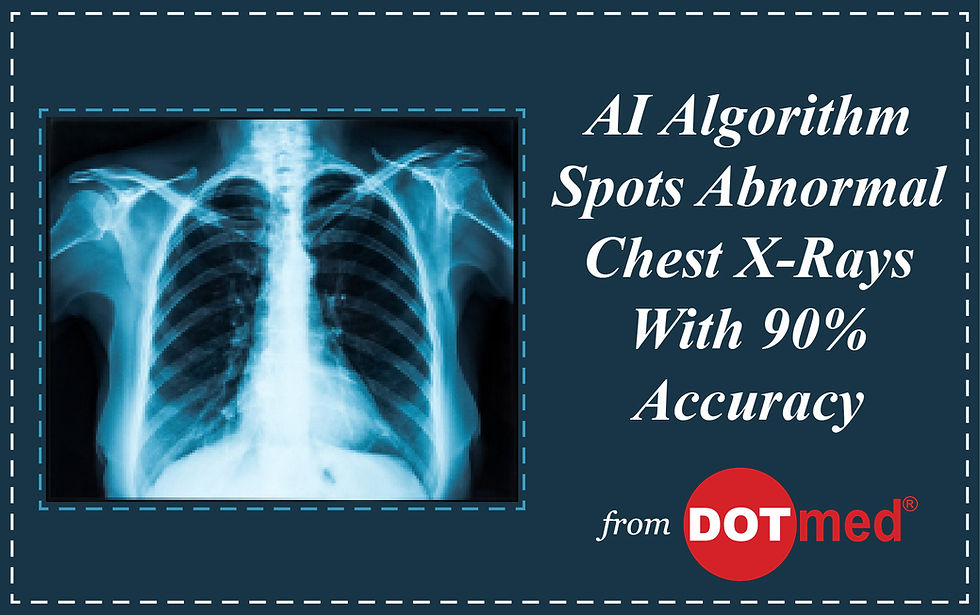
"The chest X-ray is a valuable health screening tool and a vital component of public health programs worldwide. The enormous volume produced each year creates an ever-increasing demand for radiologists," Dr. Shalini Govil, quality controller and senior adviser for the Columbia Asia Radiology Group, which took part in the study, said in a statement, noting that, "unfortunately, numerous chest X-rays displaying significant pathology are left neglected in piles of backlogs due to a lack of available radiologists to report them. Through semi-automation of the reporting process, AI can significantly reduce a radiologist's workload, improve report accuracy, reduce turnaround time and save lives.”
The study found that a deep learning algorithm trained on a large quantity of labelled X-rays can independently, and most importantly, accurately detect abnormalities on chest X-rays, according to Dr. Sundeep Reddivari, general manager, telemedicine at Columbia Asia Hospitals. The qXR algorithm was able to detect 15 chest X-ray abnormalities “at near-radiologist levels, with more than 90 percent accuracy,” according to the researchers. Qure.ai's algorithms were “tuned” on a large set of 1.2 million X-rays and were able to digest both the X-rays and corresponding radiology reports to spot abnormalities, including blunted costophrenic angle, calcification, cardiomegaly, cavity, consolidation, fibrosis, hilar enlargement, opacity and pleural effusion. The system was then put against a panel of three human radiologists in a review of 2,000 anonymized X-rays from the Columbia Asia Hospital archives. This effort also used the largest training data set ever for a chest X-ray AI, according to Prashant Warier, CEO and co-founder, Qure.ai, noting that, its 2,000 X-ray man-machine face-off is also the biggest validation study to date. “This is an exciting time for deep learning technologies in medicine,” said Warier. “As these systems increase in accuracy, so will the viability of using deep learning to extend the reach of chest X-ray interpretation, improve reporting efficiency and save lives.” In April, Qure.ai launched another deep learning algorithm, qER, that can, in ten seconds, spot brain bleeds, skull fractures and other critical head trauma in CT scans with more than 95 percent accuracy.
“The main advantage for radiologists is that AI enables automated prioritization or highlighting of patients who need urgent attention,” Pooja Rao, R&D head and co-founder of Qure.ai, told HCB News. “The radiology work list can often be long, especially at night, and this tool can help direct radiologists’ attention to critical cases. The highlighting and quantification of abnormalities both save time for the radiologist.” In May, the startup entered into a three-way partnership combining machine learning and telemedicine, when it agreed to integrate its chest X-ray technology with health IT player Telerad Tech’s RADSpa (T2), a proprietary RIS PACS platform provided worldwide by the service, Teleradiology Solutions (TRS), speeding up the return of diagnoses of X-ray and CT scans at a reduced cost.
“Teleradiology improves patient care by allowing radiologists to provide services remotely. This is particularly important when a subspecialist is needed,” Warier told HCB News. “Unfortunately, teleradiology cannot solve the fact that imaging procedures are growing approximately 15 percent annually against an increase of only 2 percent in the radiologist population. AI can alleviate this problem by improving the productivity of radiologists through screening and prioritizing cases and providing a first draft report to radiologists.”
Comments